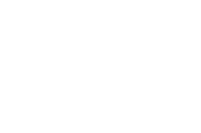
Citroën C5 Aircross: Largest Stellantis Screen
“`html Citroen Unveils New C5 Aircross SUV With Electric, hybrid Options RENNES, France — citroen has officially revealed the next generation of its C5 Aircross
“`html Citroen Unveils New C5 Aircross SUV With Electric, hybrid Options RENNES, France — citroen has officially revealed the next generation of its C5 Aircross
SpaceX Achieves Double Launch Feat with Starlink Mission from Kennedy Space Center By Archyde News Service April 28,2025 CAPE CANAVERAL,Fla. — SpaceX completed its second
Beyoncé Launches Highly Anticipated “Cowboy Carter” Tour Table of Contents 1. Beyoncé Launches Highly Anticipated “Cowboy Carter” Tour 2. “Cowboy Carter” Tour Setlist: Los Angeles,
“`html Cyberattack Paralyzes Berlin Administration Websites, Raising Security Concerns A widespread cyberattack has crippled key online services of the Berlin administration, prompting renewed calls for
“`html Citroen Unveils New C5 Aircross SUV With Electric, hybrid Options RENNES, France — citroen has officially revealed the next generation of its C5 Aircross
SpaceX Achieves Double Launch Feat with Starlink Mission from Kennedy Space Center By Archyde News Service April 28,2025 CAPE CANAVERAL,Fla. — SpaceX completed its second
Beyoncé Launches Highly Anticipated “Cowboy Carter” Tour Table of Contents 1. Beyoncé Launches Highly Anticipated “Cowboy Carter” Tour 2. “Cowboy Carter” Tour Setlist: Los Angeles,
“`html Cyberattack Paralyzes Berlin Administration Websites, Raising Security Concerns A widespread cyberattack has crippled key online services of the Berlin administration, prompting renewed calls for
© 2025 All rights reserved