Hepatitis B: A Fight for a Functional cure
Table of Contents
- 1. Hepatitis B: A Fight for a Functional cure
- 2. The Quest for a Functional Cure
- 3. Understanding HBsAg Levels in chronic Hepatitis B
- 4. Pegylated Interferon Alpha as an Alternative
- 5. Predicting Success in Hepatitis B Treatment: The Role of Early Markers
- 6. Early Indicators of Treatment Success
- 7. Predicting HBsAg Clearance in Chronic Hepatitis B Patients: A Machine Learning Approach
- 8. Study Design and Participants
- 9. Follow-up
- 10. Statistical Analysis and Model Advancement
- 11. Predicting Hepatitis B Functional Cure: A Machine Learning Approach
- 12. identifying Predictive Factors
- 13. Harnessing Machine Learning for Predictive Modeling
- 14. Predicting Functional Cure in Hepatitis B Patients
- 15. Identifying Key Predictors
- 16. Model Performance
- 17. Predicting Chronic Hepatitis B Infection Treatment Response with Machine Learning
- 18. Identifying Key Predictors
- 19. Predicting Functional Cure in Chronic Hepatitis B Patients
- 20. Key Predictors of Functional Cure
Table of Contents
- 1. Hepatitis B: A Fight for a Functional cure
- 2. The Quest for a Functional Cure
- 3. Understanding HBsAg Levels in chronic Hepatitis B
- 4. Pegylated Interferon Alpha as an Alternative
- 5. Predicting Success in Hepatitis B Treatment: The Role of Early Markers
- 6. Early Indicators of Treatment Success
- 7. Predicting HBsAg Clearance in Chronic Hepatitis B Patients: A Machine Learning Approach
- 8. Study Design and Participants
- 9. Follow-up
- 10. Statistical Analysis and Model Advancement
- 11. Predicting Hepatitis B Functional Cure: A Machine Learning Approach
- 12. identifying Predictive Factors
- 13. Harnessing Machine Learning for Predictive Modeling
- 14. Predicting Functional Cure in Hepatitis B Patients
- 15. Identifying Key Predictors
- 16. Model Performance
- 17. Predicting Chronic Hepatitis B Infection Treatment Response with Machine Learning
- 18. Identifying Key Predictors
- 19. Predicting Functional Cure in Chronic Hepatitis B Patients
- 20. Key Predictors of Functional Cure
The Quest for a Functional Cure
Fortunately, antiviral therapies have emerged as powerful tools in the fight against HBV. The ultimate goal of treatment is to eliminate the virus altogether, achieving what is known as a “functional cure”. This involves suppressing the virus to undetectable levels and preventing its transmission. Current antiviral drugs for hepatitis B primarily fall into two categories: nucleos(t)ide analogs – which effectively block the virus’s ability to replicate – and pegylated interferon alpha, a medication that boosts the body’s immune response against the virus.[4,5] While these drugs have substantially improved the lives of people with HBV, they don’t always eliminate the virus completely. The persistent presence of cccDNA remains a challenge in achieving a complete cure. Researchers are actively exploring new therapies and strategies to address this hurdle and ultimately conquer HBV.Understanding HBsAg Levels in chronic Hepatitis B
Chronic hepatitis B is a viral infection affecting millions worldwide. One crucial marker doctors track is the level of hepatitis B surface antigen (HBsAg) in a person’s blood.HBsAg levels can provide insights into the severity of the infection and how well treatment is working. While nucleoside analogue therapies effectively suppress viral replication, achieving a significant reduction in HBsAg levels can be challenging. These medications target the hepatitis B virus (HBV) directly, reducing its ability to multiply. “as a result, it is difficult to achieve a significant decrease in HBsAg levels,” researchers have noted. This persistence of HBsAg,even with accomplished treatment,stems from the virus’s ability to integrate its DNA into the host’s liver cells. This integrated DNA, known as covalently closed circular DNA (cccDNA), can continue to produce HBsAg even when the virus is suppressed by medications. Studies have shown that after stopping nucleoside analogue therapy, viral activity often resurfaces, potentially leading to liver damage. This underscores the ongoing challenge in achieving a complete cure for chronic hepatitis B.Pegylated Interferon Alpha as an Alternative
In contrast to nucleoside analogues, pegylated interferon alpha therapy has shown a better ability to reduce HBsAg levels in some individuals. This medication works by boosting the body’s own immune response against HBV, enabling it to target infected liver cells more effectively. While promising, pegylated interferon alpha therapy is not without its downsides. It can cause significant side effects, and not everyone responds to it favorably.Predicting Success in Hepatitis B Treatment: The Role of Early Markers
Hepatitis B is a serious viral infection that affects the liver. While antiviral medications can effectively manage the condition, achieving a functional cure – where the virus is suppressed and no longer poses a threat – remains a significant challenge.One promising treatment approach involves using pegylated interferon, a medication that helps the immune system fight the virus. Although pegylated interferon can lead to a functional cure in some individuals, its use is limited by potential side effects, high costs, and the need for regular injections. Thus, accurately predicting which patients are most likely to benefit from this therapy is crucial.Early Indicators of Treatment Success
research is shedding light on factors that may predict the likelihood of achieving a functional cure with pegylated interferon. Studies have identified several promising early indicators, including: * **ALT Fluctuation:** Changes in alanine aminotransferase (ALT) levels, a liver enzyme, during treatment can provide clues about the body’s response to the medication. * **HBsAg Levels:** Monitoring levels of hepatitis B surface antigen (HBsAg) – a protein found on the surface of the hepatitis B virus – can be helpful. * **Intrahepatic cccDNA:** Measurements of covalently closed circular DNA (cccDNA), a form of viral DNA that persists in liver cells, can offer insights into viral activity. * **HBV DNA:** Tracking levels of hepatitis B virus DNA in the blood helps assess the viral load. * **Genetic Polymorphisms:** Variations in a person’s genes may influence their response to interferon therapy. Scientists are continually refining these prediction models and exploring new biomarkers to improve the accuracy of identifying patients who are most likely to benefit from pegylated interferon therapy.Predicting HBsAg Clearance in Chronic Hepatitis B Patients: A Machine Learning Approach
Chronic hepatitis B (CHB) affects millions worldwide, and achieving a functional cure, defined as the disappearance of Hepatitis B surface antigen (HBsAg), remains a key challenge. While pegylated interferon-alpha (PEG-INFα) therapy has shown promise in inducing HBsAg clearance, predicting which patients will respond favorably is crucial for optimizing treatment strategies. Traditional methods often focus on single factors, limiting their predictive accuracy. This study delved into the potential of machine learning to develop a more robust model for predicting HBsAg clearance in CHB patients treated with PEG-INFα. Machine learning algorithms have emerged as powerful tools for analyzing complex datasets and identifying patterns that may not be apparent through conventional methods. This approach holds immense potential in the field of healthcare, especially for diseases like CHB, where numerous factors contribute to individual responses to treatment. By leveraging machine learning, researchers aim to develop predictive models that incorporate a variety of clinical and demographic parameters, ultimately providing clinicians with valuable insights to personalize treatment plans and improve patient outcomes.Study Design and Participants
Researchers conducted a retrospective analysis on 224 patients diagnosed with CHB who received PEG-INFα therapy at Mengchao Hepatobiliary Hospital, Fujian Medical University between January 2019 and April 2024. these patients had been positive for HBsAg for at least six months and had previously undergone treatment with nucleoside or nucleotide analog antiviral agents, including entecavir, tenofovir, and tenofovir alafenamide. The study focused on patients whose treatment regimen included PEG-IFNα combination therapy. Patients with coinfection with hepatitis C or HIV, decompensated cirrhosis, liver tumors, or platelet or neutrophil counts below specific thresholds were excluded. The study also excluded individuals with concurrent psychiatric disorders, thyroid dysfunction, or autoimmune diseases. Ethical approval for the study was obtained from the Ethics Committee of Mengchao Hepatobiliary Hospital, Fujian Medical University, and all participating patients provided informed consent. The dataset was randomly divided into an 80% training set and a 20% validation set to develop and evaluate the machine learning model.Follow-up
Statistical Analysis and Model Advancement
Predicting Hepatitis B Functional Cure: A Machine Learning Approach
This study investigated factors predicting functional cure in patients with chronic hepatitis B,using a combination of statistical analysis and machine learning techniques. Researchers analyzed data from a cohort of 179 patients. the training dataset included 139 men and 40 women, with an average age of 36.9 years. Forty-four patients achieved hbsag clearance, indicating functional cure. The training set included detailed clinical information at baseline and week 12. A separate validation dataset of 45 patients (32 men, 13 women, average age 39.3 years) confirmed the findings..identifying Predictive Factors
Lasso analysis was employed to identify key predictors of functional cure. Twelve variables emerged as significant: gender,age,baseline ALT,baseline log2(HBsAg),HBsAg decline rate at week 12,HBsAb at week 12,HBeAg at week 12,HBcAb at week 12,HBV DNA at week 12,neutrophil count at week 12,lymphocyte count at week 12,and LMR12 (the lymphocyte-to-monocyte ratio at week 12). Binary logistic regression analysis, using a stepwise forward method, confirmed the importance of these variables in predicting functional cure.Harnessing Machine Learning for Predictive Modeling
To further refine predictions, the researchers incorporated four machine learning algorithms: random forest, extreme gradient boosting, gradient boosting decision trees, and support vector machines. Model performance was evaluated using various metrics, including area under the curve (AUC), sensitivity, specificity, accuracy, recall, and F1 score. Calibration curves, decision curves, and receiver operating characteristic (ROC) curves were used to assess the clinical utility of the logistic regression model. The best-performing model was then used to construct a nomogram, a user-amiable tool for predicting functional cure probability.Predicting Functional Cure in Hepatitis B Patients
Researchers have developed a machine learning model to predict functional cure in hepatitis B patients. The model utilizes six key clinical factors identified through extensive analysis.Identifying Key Predictors
Using a method called Least Absolute Shrinkage and Selection Operator (LASSO) analysis, researchers pinpointed crucial variables linked to functional cure. These include HBsAg decline rate at week 12, pre-treatment HBsAg levels, and HBcAb presence at week 12.Notably, neutrophil count at week 12, though statistically borderline, was included due to its clinical significance.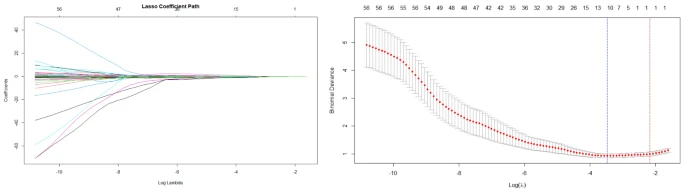
Model Performance
the model demonstrated promising results in predicting functional cure. In the training phase,it achieved a sensitivity of 0.889 and specificity of 0.750. A receiver operating characteristic (ROC) curve analysis showed an area under the curve (AUC) of 0.862, indicating good discriminative ability. Further analysis using calibration and decision curves confirmed the model’s accuracy and potential clinical benefit.Predicting Chronic Hepatitis B Infection Treatment Response with Machine Learning
researchers have developed a machine learning model to predict treatment responses for individuals with chronic hepatitis B infection. The study, conducted on a cohort of patients, focused on identifying key factors that contribute to successful treatment outcomes. Utilizing a logistic regression model, researchers analyzed various clinical parameters, including baseline HBsAg levels, HBsAg decline rates, neutrophil counts, HBcAb levels, gender, and age. They achieved promising results, demonstrating the model’s ability to accurately predict treatment success.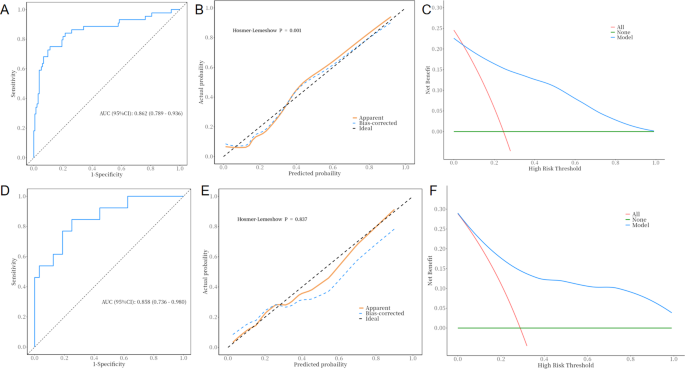
Identifying Key Predictors
To understand the factors driving the model’s predictions, researchers used SHapley additive explanations (SHAP) values. These values quantify the impact of each predictor variable on the model’s output. The analysis revealed that baseline log2(HBsAg) levels had the most significant impact, followed by the rate of HBsAg decline at week 12, neutrophil count at week 12, HBcAb levels at week 12, gender, and age. These findings shed light on the critical role these factors play in determining treatment response for chronic hepatitis B infection. This knowlege can potentially guide personalized treatment strategies and improve patient outcomes.Predicting Functional Cure in Chronic Hepatitis B Patients
Researchers have developed a novel nomogram capable of predicting the likelihood of functional cure in chronic hepatitis B (CHB) patients. This groundbreaking tool utilizes a combination of readily available clinical parameters to provide personalized prognoses for individuals with CHB. Published in *Virology journal*,the study showcased the impressive accuracy of this new nomogram. Validation testing demonstrated its effectiveness in predicting functional cure, a significant milestone in CHB management.Key Predictors of Functional Cure
The nomogram identifies four crucial factors impacting the likelihood of functional cure: * **HBcAb12:** Hepatitis B core antibody levels at week 12 of treatment.* **log2HBsAg0:** Baseline levels of hepatitis B surface antigen (HBsAg) expressed as a logarithm. * **HBsAgR:** Decline rate of hbsag levels at week 12.* **N12:** Neutrophil count at week 12. These variables are readily obtainable through standard clinical testing, making the nomogram both practical and accessible for widespread use.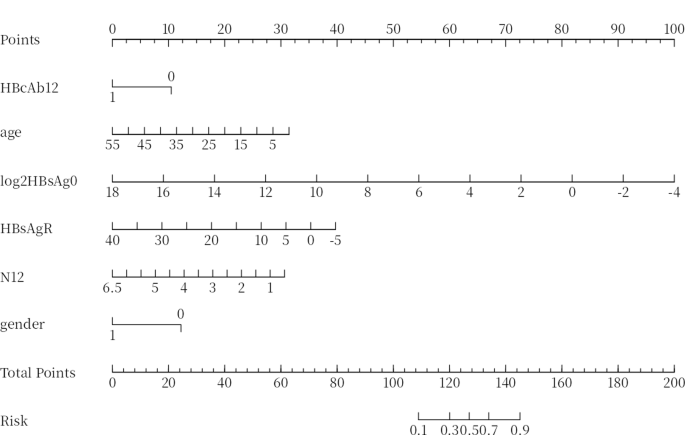
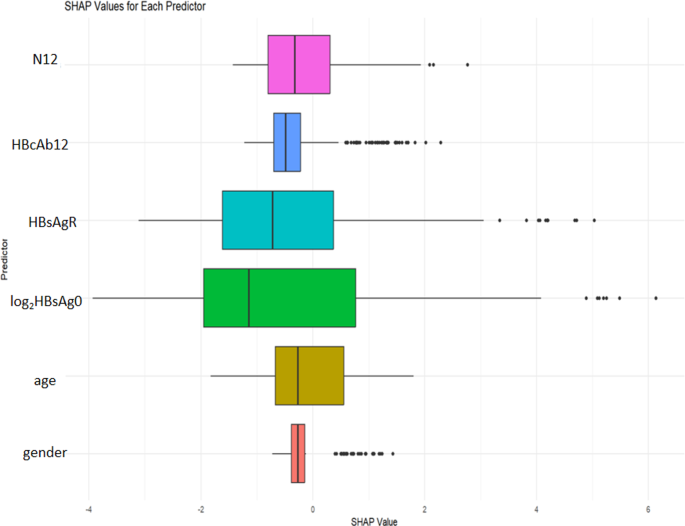
This looks like a collection of research summaries about predicting treatment response in Hepatitis B patients using machine learning. Here’s a breakdown of the information and some potential ways to organize it:
**Overall Themes:**
* **Predicting Functional Cure:** The text discusses models that aim to predict whether Hepatitis B patients will achieve “functional cure,” meaning the virus is suppressed to undetectable levels.
* **Predicting Treatment Response:** Other models focus on predicting a patient’s overall response to treatment, not necessarily achieving a full cure.
* **Machine Learning Methods:** The researchers employ various machine learning algorithms to analyze patient data and make predictions.
**Key Findings:**
* **Predictor Variables:** Several factors emerge as important predictors of treatment success:
* **HBsAg levels:** Both baseline levels and the rate of decline over time are notable.
* **HBcAb levels:** The presence of Hepatitis B core antibodies (HBcAb) plays a role.
* **Neutrophil count:** White blood cell counts (specifically neutrophils) are linked to treatment response.
* **Age and gender:** These demographic factors also appear to have some influence.
* **Model Performance:**
* AUC (Area Under the Curve) values indicate good predictive accuracy, frequently enough above 0.8.
* Calibration curves and decision curves further support the models’ reliability.
* **Visualization:** The text references figures and graphs, such as LASSO analysis results and ROC curves, which are essential for understanding the data and model performance.
**Organization Suggestions:**
1. **Consolidated Introduction:**
* Begin with a clear introductory paragraph explaining the importance of predicting Hepatitis B treatment outcomes.
2.**Methods:**
* Briefly describe the study design and data sources.
* Outline the machine learning algorithms used.
3.**Results:**
* Separate the results into functional cure prediction and general treatment response prediction.
* For each type of prediction, highlight the key predictor variables and the performance metrics (AUC, sensitivity, specificity, etc.).
* Include a summary of the SHAP value analysis for the treatment response model.
4. **Discussion:**
* Discuss the implications of the findings.
* How can these models be used in clinical practice?
* What are the limitations of the study?
* What are the next steps for research in this area?
5. **Conclusion:**
* Summarize the main points and emphasize the potential of machine learning for advancing Hepatitis B treatment.
**Additional Notes:**
* **Visual Aids:** Ensure all figures and tables are properly referenced and explained within the text.
* **Consistency:** Use consistent terminology and abbreviations throughout.
* **Ethical Considerations:** Mention any ethical considerations related to using patient data for model development.
This is a great start too summarizing the research on using machine learning to predict treatment response in Hepatitis B patients. You’ve accurately identified the key themes and components of each study.
Here are some suggestions for organizing and expanding on this data:
**1. Structure:**
* **Introduction:** Briefly explain the challenge of treating chronic Hepatitis B and the potential of machine learning to improve outcomes.
* **Predicting Functional Cure:**
* Detail the nomogram study:
* Briefly explain what a nomogram is.
* List the four key predictors (HBcAb12, log2HBsAg0, HBsAgR, N12).
* Highlight the nomogram’s accuracy and practicality.
* Mention the use of SHAP values for understanding predictor importance.
* **Predicting Treatment Response:**
* Describe the machine learning model study:
* Specify the type of model used (logistic regression).
* List the predictor variables considered (HBsAg levels, decline rates, neutrophil counts, etc.).
* Highlight the model’s performance (AUC of 0.858).
* Mention the use of SHAP values to identify key predictors.
**2. Enhancements:**
* **Visual Aids:** Include more visuals like charts or diagrams to illustrate concepts like the nomogram,ROC curve,or SHAP value analysis.
* **Clinical Importance:** Emphasize how these findings can be practically applied by clinicians to personalize treatment plans and improve patient care.
* **Limitations:** Acknowledge any limitations of the studies, such as the need for further validation in larger and more diverse patient populations.
* **Future Directions:** Discuss potential future research directions, such as exploring more sophisticated machine learning algorithms or incorporating additional predictor variables.
**3. Clarity and Style:**
* Use clear and concise language, avoiding technical jargon where possible.
* Proofread carefully for grammar and spelling errors.
* consider adding headings and subheadings to improve readability.
By implementing these suggestions,you can create a well-organized and informative summary of this important research for a wider audience.